
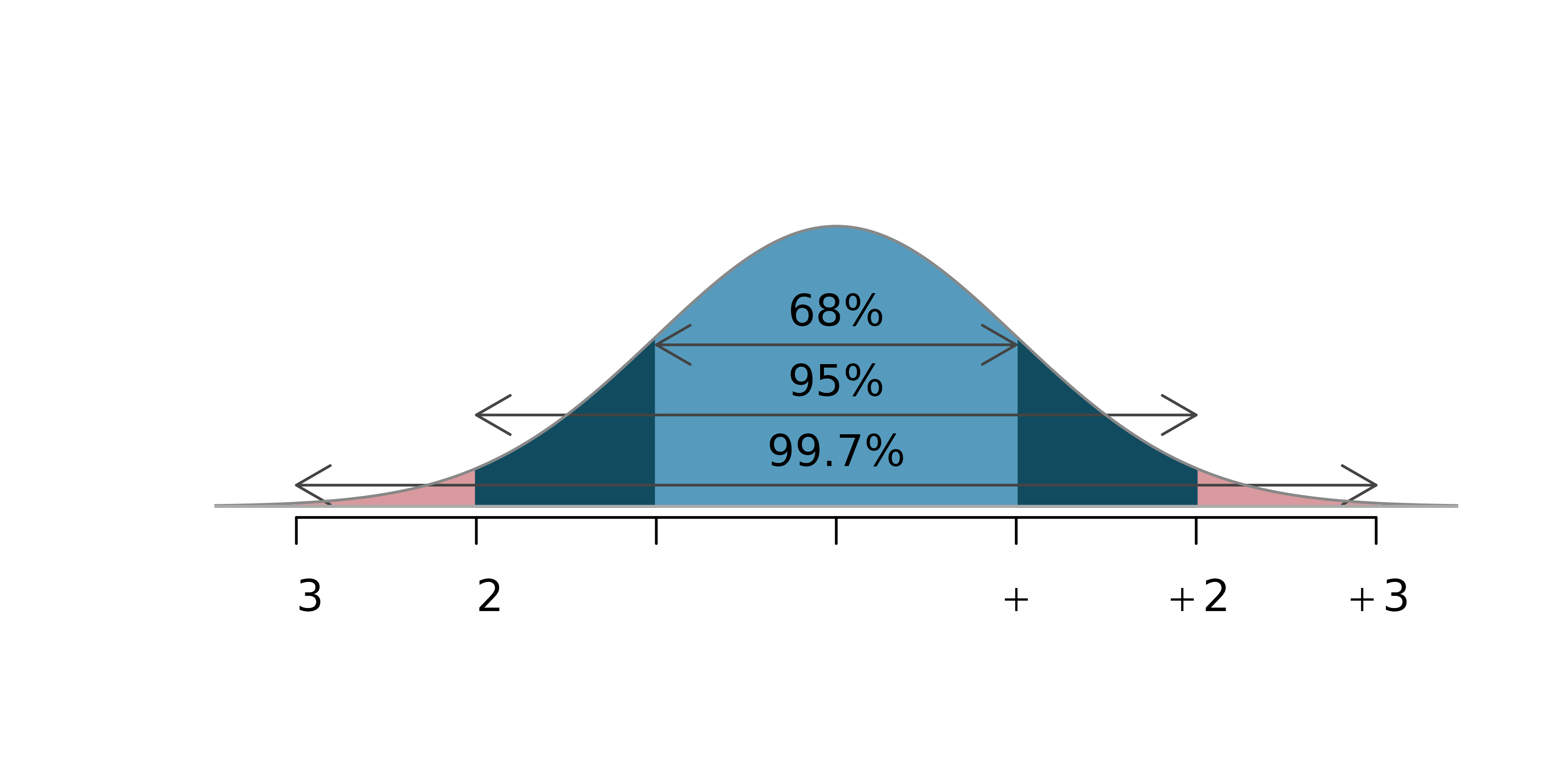
The 100 slips of paper are then put back into the large container with the other 1900 (a process called with sampling with replacement) and the container shuffled and mixed. You then draw out a sample of 100 slips of paper, calculate the mean for this sample of 100, record that mean on a piece of paper, and place it in a second smaller container. This situation can be demonstrated or simulated by recording the 2000 values on separate slips of paper and placing them in a large container.
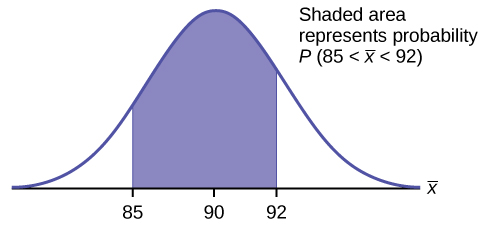
How close would you be if you only analyzed 100 specimens? Assume that the mean (µ) for the whole population is 100 mg/dl.
/CentralLimitTheoremCLT-687bdb7ec28f44539d5eabc54070058c.jpg)
This would be a lot of work, but the whole population could be tested and the true mean calculated, which would then be represented by the Greek symbol mu (µ). Blood specimens could be drawn from all 2000 patients and analyzed for glucose, for example.

A simulated experimentĬonsider the situation where there are 2000 patients available and you want to estimate the mean for that population. The values calculated from the entire population are called parameters (mu for the mean, sigma for the standard deviation), whereas the values calculated from a smaller sample are called statistics (Xbar for the mean, SD for the standard deviation). In estimating the central location of a group of test results, one could attempt to measure the entire population or to estimate the population parameters from a smaller sample. The calculation of a mean is linked to the central location or correctness of a laboratory test or method (accuracy, inaccuracy, bias, systematic error, trueness) and the calculation of an SD is often related to the dispersion or distribution of results (precision, imprecision, random error, uncertainty). In either case, individual control values should exceed the calculated control limits (expected range of values) and signal that something is wrong with the method. Changes in the method performance may cause the mean to shift the range of expected values, or cause the SD to expand the range of expected values. A common application of these statistics is the calculation of control limits to establish the range of values expected when the performance of the laboratory method is stable. The previous lesson described the calculation of the mean, SD, and CV and illustrated how these statistics can be used to describe the distribution of measurements expected from a laboratory method.
